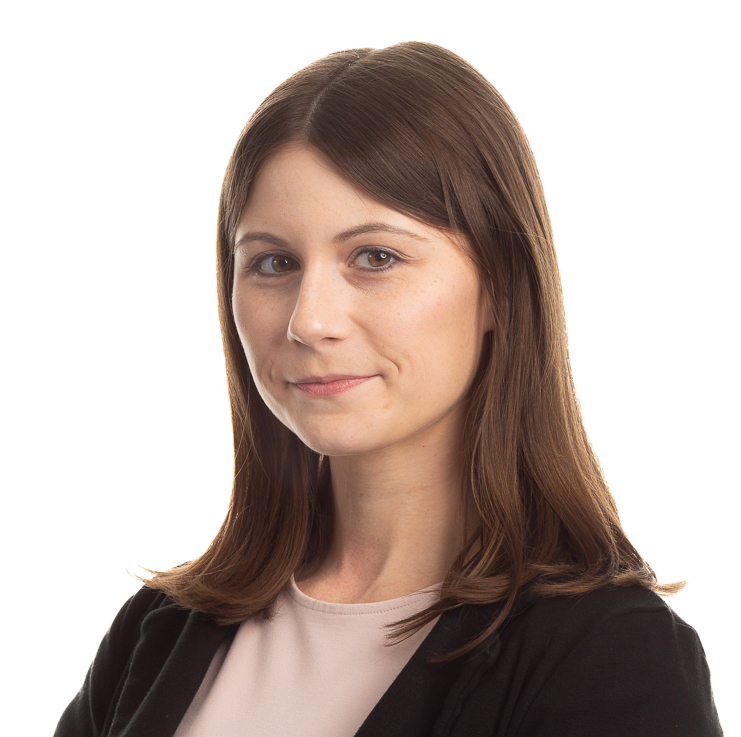
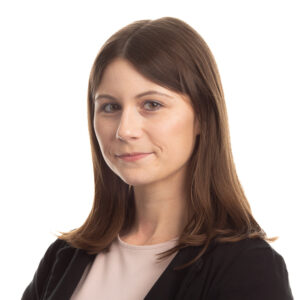
Senior Research Scientist
Forecasting of power prices is a part of everyday life for the renewables industry. Accurate power price forecasts are necessary to support the green energy transition by empowering investment, procurement, and financial-planning workflows of buyers, sellers, and investors of clean energy.
There are two primary types of models used to forecast power prices: Statistical Models and Fundamentals Models. Statistical models learn relationships between prices and other variables based on previously observed outcomes and apply these relationships to make predictions about the real world. Such models are useful in short-term, high-frequency workflows like near-term trading, where market behavior is not expected to deviate significantly from recent history. However, statistical models struggle in longer-term applications where evolving grid mixes, generation technologies, and market designs mean that the future will look different than the past. Fundamentals models can capture the impact of these changes, which is key for making long-term predictions of power prices.
Fundamentals models offer users the benefits of extendability, flexibility, and transparency in their predictions. However, the heavy computational burden of these models has traditionally required price forecasters to make simplifying assumptions about the weather. Much of the industry uses a “Weather-Normal” or “Typical Meteorological Year” to represent forward-looking weather conditions in their price models. But “atypical” meteorological conditions like heat waves, cold snaps, and severe storms can all cause dramatic surges in electricity demand, alter wind and solar supply, and affect prices. A price model that only uses a typical meteorological year will miss the extreme prices that come with extreme weather, resulting in a dramatically different modeled outcome from the true range of expected possibilities.
REsurety has taken a different, Weather-Smart approach: Our price model captures the hourly signal of 40 representative weather years in a computationally efficient way, unlocking several key benefits to customers of our fundamentals-based price models.
Key Benefits of Weather Variability in Price Models
A More Representative Mean
Weather variability is an important driver of the economics of any type of clean energy project, but storage is particularly sensitive to atypical weather conditions. For storage projects engaging in energy arbitrage, profitability relies on buying energy when prices are low and discharging it when prices are high. Energy arbitrage becomes more lucrative as the spread between high and low prices increases. Forecasting price using a weather-normal year results in inaccurate forecasts of storage value because it misses the extremes of price that drive value for storage resources. Simply put, weather-normal modeling materially undervalues the energy arbitrage revenue opportunity of storage projects.
When adequate weather variability is represented in a price model, the predicted mean value of a storage project will better reflect these high price events, as illustrated in the schematic below. Two cases are considered. The blue line represents the case with a distribution of daily forecasted storage project values created using prices produced by a Weather-Smart model fed with the signal of 40 weather years. The second case represents in green the distribution of daily storage values based on prices produced by modeling a single weather-normal year of data. While both distributions of storage project value report a similar median, the Weather-Smart distribution produces a mean value that is significantly higher than its median. In this case, the mean can serve as a simple, single quantity that distills the potential profitability of a storage project in a way that acknowledges the extreme events contributing to its value.
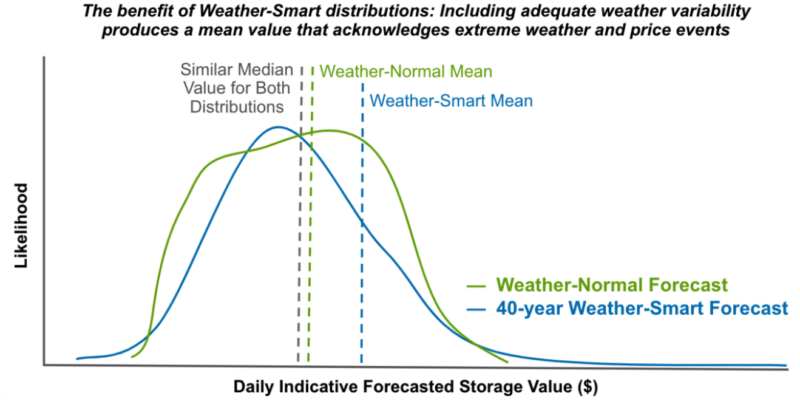
Visibility into Range
REsurety’s fundamentals price model gives users unique insight into their price forecasts, and by modeling hourly weather variability representative of 40 years, we elevate this insight to give visibility into a broad range of outcomes. For example, a forecast driven by a weather-normal year of input data will give a forecasted wind capture rate for a typical summer, perhaps at 80%. But how can a clean energy buyer set expectations with their financial planning team in the event of a warmer-than-average summer with lower-than-average wind speeds that yields a capture rate of only 60%? A wide distribution of potential outcomes exists depending on the weather conditions, and visibility into this range of outcomes is important for making financial decisions and planning for downside scenarios. This approach is outlined in greater depth in another REsurety blog post.
Better-Informed Portfolio Optimization
Visibility into range provided by Weather-Smart price forecasts lends itself to better portfolio optimization as well. Portfolio risk mitigation is possible through understanding the tails of distributions of individual assets, which price models with awareness of adequate weather variability can provide. By optimizing portfolios to include assets that provide value in countering scenarios, the overall portfolio risk can be narrowed. For example, a forecasted summer month that has low wind speeds and high temperatures will likely be profitable for solar projects, which can help offset low wind value or Fixed Volume Swap losses. A Weather-Smart price model that is aware of such anomalous weather conditions allows for this kind of portfolio optimization.
About the author
Jessica Tomaszewski is an atmospheric scientist with experience in boundary layer meteorology, numerical weather prediction, and wind resource assessment. Prior to joining REsurety, Jessica completed a National Science Foundation Graduate Research Fellowship with a focus on simulating interactions between wind farms and the lower atmosphere, as well as two summer internships at NextEra Analytics investigating improvements to the wind farm wake modeling process. As a research scientist at REsurety, she builds and investigates new techniques for analyzing renewable resources and mitigating their financial risk.
Jessica holds a PhD and Master’s degree in Atmospheric and Oceanic Sciences from the University of Colorado. She also holds a Bachelor’s degree in Meteorology from the University of Oklahoma. Learn more about Jessica here.
Return to the blog post main menu.